Image generation AI for predicting the deformation of splashing drops
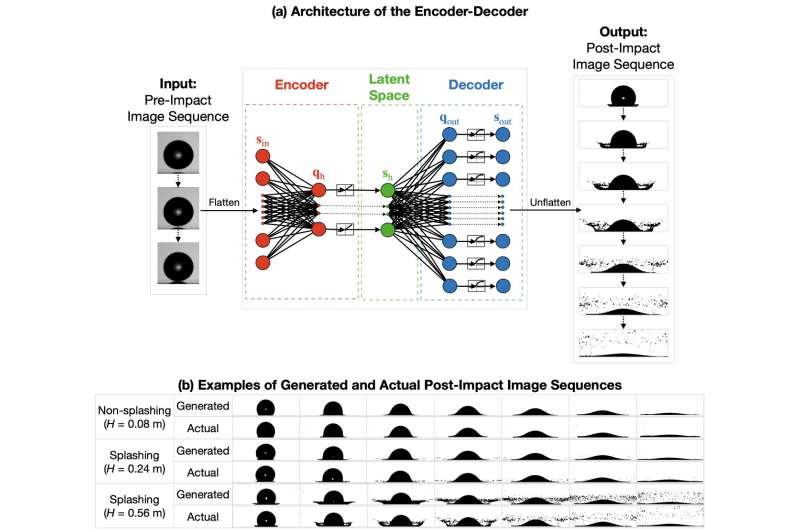
The impact of a drop on a solid surface is an important phenomenon that has various implications and applications. When the drop splashes, it can cause soil erosion, dispersal of plant pathogens, deterioration of printing and paint qualities, and many other issues. Therefore, it is necessary to predict the deformation of a splashing drop to minimize the adverse effects. However, the multiphase nature of this splashing causes complications in the prediction.
To tackle this problem, several drop-impact studies have adopted artificial intelligence (AI) models and have shown excellent performance. However, the models developed in these studies use physical parameters as inputs and outputs, so it’s difficult to capture the deformation of the impacting drop.
At the Tokyo University of Agriculture and Technology, a research team from the Department of Mechanical Systems Engineering proposed a computer-vision strategy and successfully predicted the deformation using image data. The research team led by Prof Yoshiyuki Tagawa, which includes Jingzu Yee (postdoctoral researcher), Daichi Igarashi (1st-year master’s student) and Shun Miyatake (4th-year undergraduate student), had their findings published in Machine Learning: Science and Technology.
In their research, the architecture of an encoder–decoder, which can use images as input and output, has been adopted to develop an image-based AI model to predict the drop deformation. By taking a pre-impact image sequence as the input, the trained encoder–decoder has successfully generated image sequences that show the deformation of a drop during the impact.
Remarkably, the generated image sequences are very similar to the actual image sequences captured during the experiment. The quantitative evaluation of the generated image sequences showed that in each frame of these generated image sequences, the spreading diameter of the drop was found to be in close alignment with that of the actual image sequences. Moreover, there was also a high accuracy in splashing/non-splashing prediction.
These findings demonstrate the ability of the trained encoder–decoder to generate image sequences that can accurately represent the deformation of a drop during the impact.
“The approach proposed by this research offers a faster and more cost-effective alternative to experimental and numerical studies,” said Yoshiyuki Tagawa, professor at the Tokyo University of Agriculture and Technology. “This achievement is important in understanding and minimizing the adverse effects of splashing. In addition, it has shown the great potential of using AI and machine learning for scientific studies.”
More information:
Jingzu Yee et al, Prediction of the morphological evolution of a splashing drop using an encoder–decoder, Machine Learning: Science and Technology (2023). DOI: 10.1088/2632-2153/acc727
Provided by
Tokyo University of Agriculture and Technology
Citation:
Image generation AI for predicting the deformation of splashing drops (2023, April 19)
retrieved 19 April 2023
from https://techxplore.com/news/2023-04-image-generation-ai-deformation-splashing.html
This document is subject to copyright. Apart from any fair dealing for the purpose of private study or research, no
part may be reproduced without the written permission. The content is provided for information purposes only.
For all the latest Technology News Click Here
For the latest news and updates, follow us on Google News.