Machine learning models to help photovoltaic systems find their place
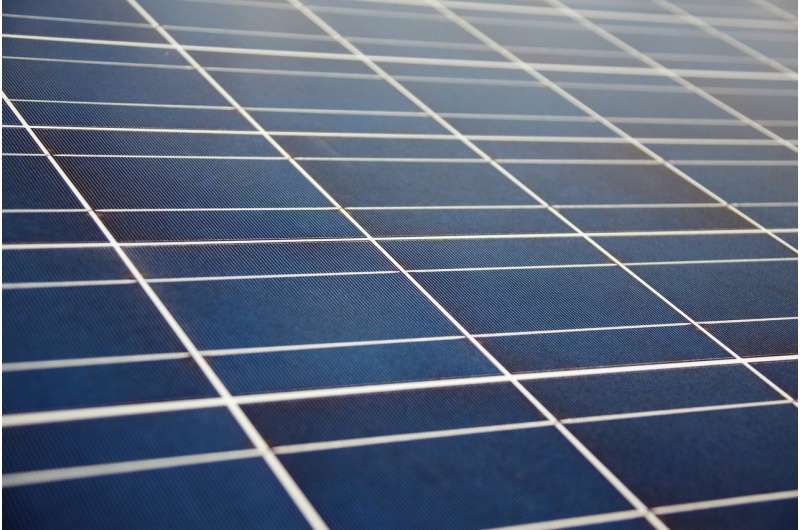
With the looming threat of climate change, it is high time we embrace renewable energy sources on a larger scale. Photovoltaic systems, which generate electricity from the nearly limitless supply of sunlight energy, are one of the most promising ways of generating clean energy. However, integrating photovoltaic systems into existing power grids is not a straightforward process. Because the power output of photovoltaic systems depends heavily on environmental conditions, power plant and grid managers need estimations of how much power will be injected by photovoltaic systems so as to plan optimal generation and maintenance schedules, among other important operational aspects.
In line with modern trends, if something needs predicting, you can safely bet that artificial intelligence will make an appearance. To date, there are many algorithms that can estimate the power produced by photovoltaic systems several hours ahead by learning from previous data and analyzing current variables. One of them, called adaptive neuro-fuzzy inference system (ANFIS), has been widely applied for forecasting the performance of complex renewable energy systems. Since its inception, many researchers have combined ANFIS with a variety of machine learning algorithms to improve its performance even further.
In a recent study published in Renewable and Sustainable Energy Reviews, a research team led by Jong Wan Hu from Incheon National University, Korea, developed two new ANFIS-based models to better estimate the power generated by photovoltaic systems ahead of time by up to a full day. These two models are ‘hybrid algorithms’ because they combine the traditional ANFIS approach with two different particle swarm optimization methods, which are powerful and computationally efficient strategies for finding optimal solutions to optimization problems.
To assess the performance of their models, the team compared them with other ANFIS-based hybrid algorithms. They tested the predictive abilities of each model using real data from an actual photovoltaic system deployed in Italy in a previous study. The results, as Dr. Hu remarks, were very promising: “One of the two models we developed outperformed all the hybrid models tested, and hence showed great potential for predicting the photovoltaic power of solar systems at both short- and long-time horizons.”
The findings of this study could have immediate implications in the field of photovoltaic systems from software and production perspectives. “In terms of software, our models can be turned into applications that accurately estimate photovoltaic system values, leading to enhanced performance and grid operation. In terms of production, our methods can translate into a direct increase in photovoltaic power by helping select variables that can be used in the photovoltaic system’s design,” explains Dr. Hu. Let us hope this work helps us in the transition to sustainable energy sources!
Research: Photovoltaics can make the world fossil-free faster than expected
Mosbeh R.Kaloop et al, Novel application of adaptive swarm intelligence techniques coupled with adaptive network-based fuzzy inference system in predicting photovoltaic power, Renewable and Sustainable Energy Reviews (2021). DOI: 10.1016/j.rser.2021.111315
Provided by
Incheon National University
Citation:
Machine learning models to help photovoltaic systems find their place (2021, July 20)
retrieved 20 July 2021
from https://techxplore.com/news/2021-07-machine-photovoltaic.html
This document is subject to copyright. Apart from any fair dealing for the purpose of private study or research, no
part may be reproduced without the written permission. The content is provided for information purposes only.
For all the latest Technology News Click Here
For the latest news and updates, follow us on Google News.