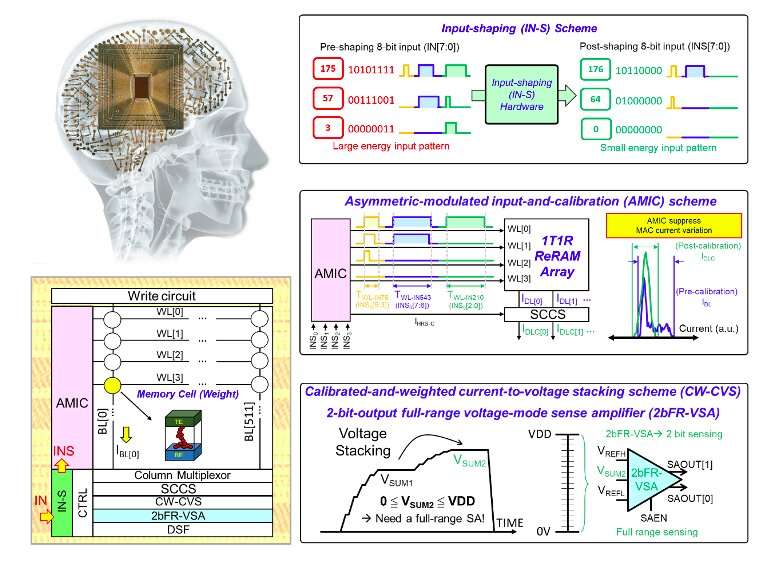
Edge AI devices, systems that combine artificial intelligence (AI) and edge computing techniques, are becoming an essential part of the rapidly growing Internet of Things (IoT) ecosystem. These devices include smart speakers, smart phones, robots, self-driven cars, drones and data-processing surveillance cameras.
While these technologies have become increasingly advanced over the past few years, most of them exhibit limited energy efficiencies, inference accuracies, and battery lifetimes. Non-volatile computing-in-memory (nvCIM) architectures, an emerging class of approaches that minimize the movement of data between processors and memory components, could help to significantly reduce the latency and energy consumption associated with complex AI computations.
Researchers at the Taiwan Semiconductor Manufacturing Company (TSMC) recently developed a new four-megabit (4Mb) nvCIM approach that could help to improve the overall performance of edge AI devices. Their proposed architecture, presented in a paper published in Nature Electronics, combines memory cells with peripheral circuitry based on complementary metal-oxide semiconductor (CMOS) technology.
“The computing latency and energy consumption of neural networks operating for AI applications using conventional von Neumann computing architectures are dominated by the movement of data between the processing element and memory, creating a performance bottleneck known as the memory wall,” Meng-Fan Chang, one of the researchers who carried out the study, told TechXplore. “NvCIM may help to overcome the memory-wall bottleneck for battery-powered AI edge devices by allowing analog operations for vector-matrix multiplication, which is the major computing operation in the neural network during the inference stage.”
NvCIM architectures can significantly reduce the amount of data that is transferred between processors and memories in AI edge devices, particularly while the devices are performing inference and power-on operations on-chip. This can in turn lead to better energy efficiencies and extended battery lifetimes.
Chang and his colleagues have been developing computing-in-memory (CIM) devices for almost 10 years. In their past studies, they used a variety of different memory components, including SRAM, STT-MRAM, PCM, ReRAM, and NAND-Flash, to assess the resulting performance.
“Over the past five years, we presented 40 papers related to CIM at top Microelectronics conferences (ISSCC, IEDM and DAC),” Chang explained. “Our recent work builds on our long-term research on CIM, which outlined technical background of memory circuit design, the system-level chip design of neural networks, and AI algorithms.”
The new 4Mb nvCIM architecture created by the researchers is based on 22-nm-foundry resistive random-access memory (ReRAM) devices, also known as memristors. Remarkably, Chang and his colleagues found that it can perform high-precision dot-product operations involving an 8-bit input, 8-bit weight and 14-bit output with little latency and high energy efficiencies.
“We developed a hardware-based input-shaping circuit, using software-hardware co-design methods to improve energy efficiency without degrading the system-level inference accuracy,” Chang said. “To reduce computing latency and improve readout accuracy, we develop an asymmetrically modulated input-and-calibration (AMIC) scheme.”
To reduce their device’s computing latency, the researchers built a calibrated and weighted current-to-voltage stacking circuit with a 2-bit output and full-range voltage-mode sense amplifier. This circuit also ensures a good readout yield for the most significant bits (MSBs), reducing the architecture’s overall readout energy.
The architecture created by Chang and his colleagues can tackle complex computing tasks across a variety of application scenarios. In addition, compared to other nvCIM architectures proposed in the past, it is more precise, has a higher computing throughput and a larger memory capacity, consumes less energy, and has a lower computing latency.
“We also focused on software-hardware co-design to further improve the chip-level performance,” Chang said. “Existing advanced edge devices for AI and AI-enabled Internet of Things (AIoT) applications commonly adopt nvCIM for power-off data storage to suppress power consumption in standby mode and light computing tasks during wake-up.”
In the future, the architecture developed by this team of researchers could be used to enhance the performance and energy efficiency of different edge AI devices, ranging from smart phones to more sophisticated robotic systems. Among other things, it can support basic vector-matrix multiplications (VMMs) performed by various neural network models, including convolution neural networks (CNNs) for image classification or deep neural network (DNNs).
“Circuit level optimization, nvCIM architecture novelty, improvement of specification, and performance of nvCIM macro are definitely next on our roadmap,” Chang added. “Software-hardware co-design is also one of our future research topics, we aim at developing nvCIM-friendly neural network algorithms to further maximize the performance of nvCIM macro. Beyond that, our goal is to integrate the nvCIM macro and other necessary digital circuits into a chip-level system design for the next generation AI chips.”
World’s first MRAM-based in-memory computing
Je-Min Hung et al, A four-megabit compute-in-memory macro with eight-bit precision based on CMOS and resistive random-access memory for AI edge devices, Nature Electronics (2021). DOI: 10.1038/s41928-021-00676-9
© 2022 Science X Network
Citation:
A four-megabit nvCIM macro for edge AI devices (2022, January 27)
retrieved 27 January 2022
from https://techxplore.com/news/2022-01-four-megabit-nvcim-macro-edge-ai.html
This document is subject to copyright. Apart from any fair dealing for the purpose of private study or research, no
part may be reproduced without the written permission. The content is provided for information purposes only.
For all the latest Technology News Click Here
For the latest news and updates, follow us on Google News.