Beyond the ‘black box’: Toward AI that is both generative and explainable
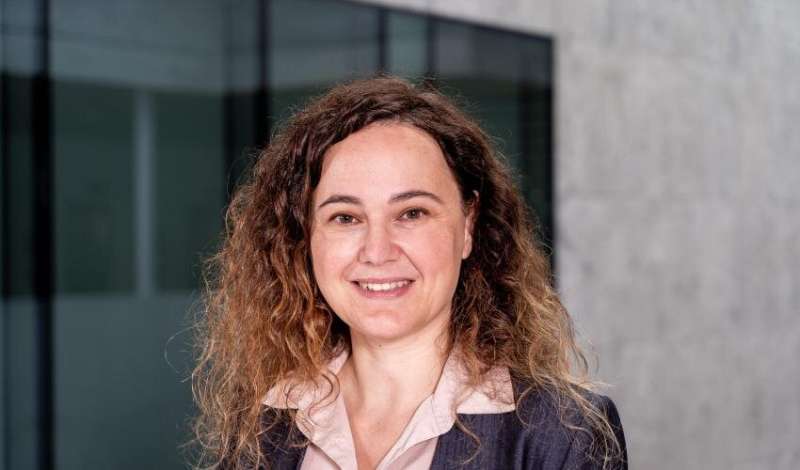
The latest breakthroughs in artificial intelligence (AI) build on deep neural networks, a specific type of AI system. Today, their applications are known to the general public in several areas, in particular, the so-called large language models capable of producing human-resembling text and conversations (ChatGPT, Bing AI, Bard) and the text-to-image generative models which can produce striking images from a text captions (DALL-E 2, Imagen, Stable Diffusion, Midjourney), as well as others connected to the recognition, production and translation of speech and sound.
What is behind the success of these techniques? The domains in which those successes happened are not haphazard or random, but rather perfectly suited for the strengths of neural networks as they exist today. Indeed, neural networks are proficient at emulating complex actions, even when they are difficult to define in precise terms, as long as we can feed them enormous amounts of examples to learn from. For example, it is challenging to define a “beautiful” or even “well-formed” image, sound or text, in precise mathematical terms. We do have, however, copious amounts of each to learn from.
A big challenge that AI is currently facing in its path to broaden its range of applications, however, is that deep neural networks are inscrutable. Indeed, the underpinnings of current AI systems are essentially a gigantic table of numbers. Those are not just any random numbers: the AI systems find the numbers that allow them to best reproduce the examples they have learned from. However, the table’s size and composition become so complex that they are devoid of any real semantic structure. As a result, we understand how neural networks function only in a superficial sense: we could crunch the numbers and reproduce any output from a given output.
But we know pretty much nothing beyond that. For this reason, they are called black-box systems, notoriously unable to provide a justification for their output. This can be fine for some image, text or sound generation: one ugly or malformed piece does not jeopardize the usefulness of the tool if many others are good. However, it might be decidedly inappropriate for political, ethical, financial or business decisions: here one wants to be able to explain why a certain decision is considered to be the right one. Furthermore, emulating past decision processes without having/understanding the rationale behind it might make us overlook possible biases or latent errors.
In order to widen the applicability of AI, we should thus adopt algorithms that provide a justification for their answers (“explainable AI”). For neural networks, this involve complementing the massive engineering efforts of the last years (which brought on the recent stunning results) with a deeper understanding of their structure from a mathematical and theoretical perspective. Mathematical optimization approaches are a privileged route towards bridging this gap in understanding.
On its own, optimization is the key instrument for solving many operations problems. Roughly speaking, it deals with selecting the best solution for a given problem, out of a set of possible ones. The crucial point is formally proving (i.e., explaining) optimality of a given solution without enumerating all possibilities, but rather by exploiting the mathematical structure of the problem under consideration. Unsurprisingly, optimization is a pillar for the mathematical foundation of modern AI.
Indeed, such systems deal with finding the algorithms and parameters that best model a given task, and this, in itself, can be seen as an optimization problem. Furthermore, optimization problems are often formalized as maximizing an objective function subject to given constraints, where both the objective and the constraints are specified in terms that can be readily understood by humans. This is why strengthening the interplay of AI systems and optimization techniques can help in breaking their inscrutable nature. It is there that the amalgamation of optimization and AI bears its most promising fruits.
Provided by
Bocconi University
Citation:
Beyond the ‘black box’: Toward AI that is both generative and explainable (2023, March 23)
retrieved 23 March 2023
from https://techxplore.com/news/2023-03-black-ai-generative.html
This document is subject to copyright. Apart from any fair dealing for the purpose of private study or research, no
part may be reproduced without the written permission. The content is provided for information purposes only.
For all the latest Technology News Click Here
For the latest news and updates, follow us on Google News.