Novel system uses reinforcement learning to teach robotic cars to speed
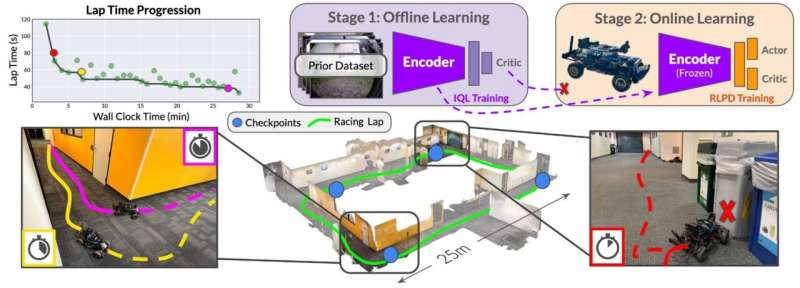
Fast cars. Millions of us love them. The idea transcends national borders, race, religion, politics. We embraced them for more than a century, beginning in the early 1900s with the stately Stutz Bearcat and Mercer Raceabout (known as “the Steinway of the automobile world”), to the sexy Pontiac GTOs and Ford Mustangs of the 1960s, and through to the ultimate luxury creations of the Lamborghini and Ferrari families.
“Transformers” film director Michael Bay, who knows a thing or two about outrageous vehicles, has declared, “Fast cars are my only vice.” Many would agree.
Die-hard racing fans would also heartily endorse award-winning race car driver Parnelli Jones’s assessment of life in the fast lane: “If you’re in control, you’re not going fast enough.”
Now, robotic cars are joining in on the fun.
Researchers at the University of California at Berkeley have developed what they say is the first system that trains small-scale robotic cars to autonomously engage in high-speed driving while adapting to and improving in real world environments.
“Our system, FastRLAP, trains autonomously in the real world without human interventions, and without requiring any simulation or expert demonstrations,” said graduate student robotics researcher Kyle Stachowicz.
He outlined components he and his team used in their research, now available on the arXiv preprint server. First is the initialization stage that generates data about different driving environments. A model car is manually directed along varying courses where its primary goal is collision avoidance, not speed. The vehicle does not need to be the same one that ultimately learns to drive fast.
Once a large dataset covering a broad range of routes is compiled, a robotic car is deployed on a course it needs to learn. A preliminary lap is made so a perimeter can be defined, and then the car its on its own. With the dataset in hand, the car is trained via RL (reinforcement learning) algorithms to navigate the course more efficiently over time, avoiding obstacles, and increasing its efficiency through directional and speed adjustments.
Researchers said they were “surprised” to find that the robotic cars could learn to speed through racing courses with less than 20 minutes of training.
According to Stachowicz, the results “exhibit emergent aggressive driving skills, such as timing braking and acceleration around turns and avoiding areas that impede the robot’s motion.” The skill exhibited by the robotic car “approaches the performance of a human driver using a similar first-person interface over the course of training.”
An example of a skill learned by the vehicle is the idea of the “racing line.”
The robotic car finds “a smooth path through the lap … maximizing its speed through tight corners,” Stachowicz said. “The robot learns to carry its speed into the apex, then brakes sharply to turn and accelerates out of the corner, to minimize the driving duration.”
In another example, the vehicle learns to oversteer slightly when turning on a low-friction surface, “drifting into the corner to achieve fast rotation without braking during the turn.”
Stachowicz said the system will need to address issues of safety in the future. Currently, collision avoidance is rewarded simply because it prevents task failure. It doesn’t resort to safety measures such as proceeding cautiously in unfamiliar environments.
“We hope that addressing these limitations will enable RL-based systems to learn complex and highly performant navigation skills in a wide range of domains, and we believe that our work can provide a stepping stone toward this,” he said.
Like Tom Cruise’s “Maverick” character in “Top Gun,” the researchers “feel the need, the need for speed.” So far, they’re on the right track.
More information:
Kyle Stachowicz et al, FastRLAP: A System for Learning High-Speed Driving via Deep RL and Autonomous Practicing, arXiv (2023). DOI: 10.48550/arxiv.2304.09831
Project site: sites.google.com/view/fastrlap?pli=1
© 2023 Science X Network
Citation:
Novel system uses reinforcement learning to teach robotic cars to speed (2023, May 1)
retrieved 1 May 2023
from https://techxplore.com/news/2023-05-robotic-cars.html
This document is subject to copyright. Apart from any fair dealing for the purpose of private study or research, no
part may be reproduced without the written permission. The content is provided for information purposes only.
For all the latest Technology News Click Here
For the latest news and updates, follow us on Google News.