Optimizing phase change materials could reduce power plant water consumption
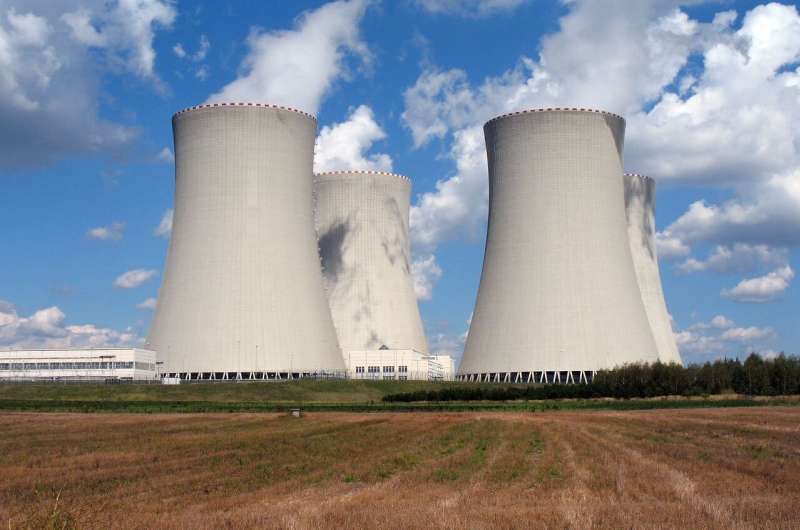
The food-water-energy nexus dictates that there is a direct link between these three necessities, and stressing one directly impacts the supply of the other two. As the population grows, human demand for energy and food has caused freshwater reserves to slowly deplete. Power plants are one of the main culprits contributing to this issue, as they use trillions of gallons of fresh water annually to prevent overheating.
A research group led by Debjyoti Banerjee, professor in the J. Mike Walker ’66 Department of Mechanical Engineering at Texas A&M University, has shown that specific phase change materials (PCMs) can cool steam turbines used in power plants, averting fresh water usage. Simultaneously, the group used machine-learning techniques to enhance the reliability and energy storage capacity of various PCM-based cooling platforms to develop powerful “cold batteries” that dispatch on demand.
The researchers’ publication, “Leveraging Machine Learning (Artificial Neural Networks) for Enhancing Performance and Reliability of Thermal Energy Storage Platforms Utilizing Phase Change Materials,” was published in the American Society of Mechanical Engineers Journal of Energy Resources Technology.
Power plants and process industries use fresh water in cooling towers to reduce costs and improve reliability. Water runs through the cooling tower, absorbing the heat and turning into vapor, which is then used to condense the steam from the turbine exhaust.
With high demands on fresh water, alternate methods like using PCMs that can morph from a solid to a liquid state by absorbing heat energy are gaining more attention for cooling power plants and process industries.
The first material the team examined was bioderived waxy materials (similar to lard): natural products with low carbon footprints that are relatively cheap. Although effective, the researchers showed that waxes (paraffins) could not store as much energy nor deliver the cooling power they originally hypothesized, therefore, not providing enough cooling for extreme climates or providing safety amid extreme weather events.
This led to testing another PCM called salt hydrates that are also inexpensive and safe for the environment. Salt hydrates pack more punch than waxes and lards, approximately harboring two to three times the amount of energy while melting at faster rates. However, these materials have a known flaw—they take too long to solidify (as they need to be “subcooled”). Without a reliable melting and freezing method, the salt hydrates are ineffective.
“Think of the process as an electric car battery—you want it to take very little time to recharge, but it needs to run for a long time,” Banerjee said. “The same concept can be applied to PCMs. We need a PCM to recharge (freeze) quickly, yet melt over long durations.”
To ramp up the reliability and speed up freezing of these PCMs, the researchers turned to machine learning. Using the readings from just three miniature temperature sensors that act like thermometers, they recorded the melting-time history. They then implemented machine learning to predict when and how much of the PCM will melt and when the freezing will start, maximizing both cooling power and capacity.
“Using this method, we found that if you melt only 90% of the salt hydrate and leave 10% solidified, then the moment you start the cooling cycle, it immediately starts freezing,” Banerjee said. “The beauty of this method is that with a bare-bones apparatus of three sensors and a simple computer program, we have created a system that is cost-effective, reliable and sustainable.”
Currently, other machine-learning algorithms require years of data to achieve this type of accuracy for power plants whereas Banerjee’s new method requires only a few days. The algorithm can tell the operator within one hour (and as much as three hours) before the system will reach the peak percentage for melting with a five to 1o-minute prediction accuracy. The technique can be retrofitted on any existing cooling unit in any process industry or power plant.
New 3D printable phase-changing composites can regulate temperatures inside buildings
Aditya Chuttar et al, Leveraging Machine Learning (Artificial Neural Networks) for Enhancing Performance and Reliability of Thermal Energy Storage Platforms Utilizing Phase Change Materials, Journal of Energy Resources Technology (2021). DOI: 10.1115/1.4051048
Citation:
Optimizing phase change materials could reduce power plant water consumption (2021, July 29)
retrieved 29 July 2021
from https://techxplore.com/news/2021-07-optimizing-phase-materials-power-consumption.html
This document is subject to copyright. Apart from any fair dealing for the purpose of private study or research, no
part may be reproduced without the written permission. The content is provided for information purposes only.
For all the latest Technology News Click Here
For the latest news and updates, follow us on Google News.