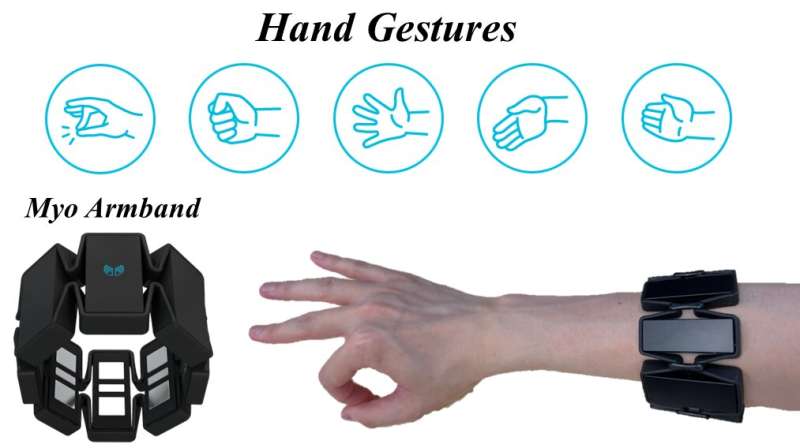
Surface electromyography (EMG) has been widely used to measure the electrical activity of muscles. However, the variability in EMG sensing signals due to biological differences of different users significantly degrades the performance and potential of EMG systems.
Recently, researchers from City University of Hong Kong (CityU) developed a deep learning-based framework called EMGSense, which can achieve high sensing performance for new users using AI self-training techniques. This opens a new path for developing more advanced and accurate wearable EMG devices in areas like neurorehabilitation and virtual reality.
This latest invention won an award at The 21st International Conference on Pervasive Computing and Communications (PerCom 2023) held at Atlanta, U.S.. It helps overcome the bottleneck in existing approaches and supports the widespread adoption of EMG-based applications.
EMG measures the electrical activity of muscles using surface electrodes on the skin. EMG-based sensing has attracted considerable attention in recent years and has created a lot of intelligent applications, such as neurorehabilitation, activity recognition, gesture recognition and virtual reality.
But a fundamental challenge in existing EMG systems is how to tackle cross-user scenarios. EMG signals can be seriously influenced by various biological factors, such as body fat, skin conditions, age and fatigue. So significant performance degradation would be caused by time-varying biological heterogeneity when the EMG system is employed by different users.
To address this challenge, researchers from the Department of Computer Science at CityU recently proposed the first low-effort, AI-empowered domain adaptation framework, called EMGSense, which provides high-accuracy EMG sensing for new users using AI-training techniques. EMGSense is a self-supervised system with a self-training AI strategy. It can cope with the performance degradation caused by inter-user biological heterogeneity.
The new framework integrates advanced self-supervised techniques into a carefully designed deep neural network (DNN) structure. It uses small-scale unlabeled data from a new user and pre-collected data from several existing users to train a discriminative model to realize intelligent applications for new users. The pre-collected data is stored in the cloud and can serve all new users, reducing the burden of data collection and annotation.
EMGSense’s DNN structure involves two training stages, which complement each other. It first eliminates user-specific features in the feature space for easy transferring, and then it employs AI techniques to re-learn new target’s user-specific biological features in that space for high-performance EMG sensing. This allows EMGSense to adapt to new users with satisfactory performance in a low-effort, self-supervised manner without wasting significant deployment overhead.
In addition, the researchers leveraged the unlabeled data collected during the usage to achieve long-term robust performance that can handle the time-varying nature of EMG signals.
A comprehensive evaluation of two sizable datasets collected from 13 participants indicated that EMGSense achieved an average accuracy of 91.9% and 81.2% in gesture recognition and activity recognition, respectively. EMGSense also outperformed state-of-the-art EMG-oriented domain adaptation approaches by 12.5%–17.4% and achieved comparable performance with one trained in a supervised-learning manner.
The novel EMGSense framework has the potential to revolutionize the field of EMG sensing by reducing the burden of data collection and annotation, while achieving high accuracy in a low-effort manner. It fills the research gap in heterogeneity problems in EMG sensing and enables a variety of novel EMG-based cross-user applications, such as clinical practice, neurorehabilitation and human-machine interaction. It also makes a humble step towards the ubiquity of smart EMG wearable devices with higher performance in real-world scenarios.
The paper is published as part of the 2023 IEEE International Conference on Pervasive Computing and Communications (PerCom).
More information:
Di Duan et al, EMGSense: A Low-Effort Self-Supervised Domain Adaptation Framework for EMG Sensing, 2023 IEEE International Conference on Pervasive Computing and Communications (PerCom) (2023). DOI: 10.1109/PERCOM56429.2023.10099164
Conference: www.percom.org/
Citation:
Researchers develop a self-supervised AI adaptation framework to enhance sensing accuracy of EMG devices (2023, May 25)
retrieved 25 May 2023
from https://techxplore.com/news/2023-05-self-supervised-ai-framework-accuracy-emg.html
This document is subject to copyright. Apart from any fair dealing for the purpose of private study or research, no
part may be reproduced without the written permission. The content is provided for information purposes only.
For all the latest Technology News Click Here
For the latest news and updates, follow us on Google News.